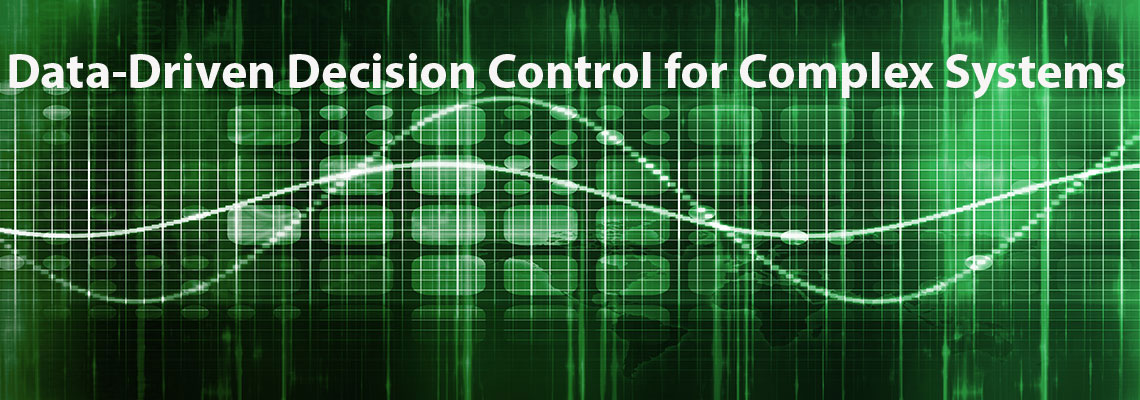
The objective of DnC2S is to develop the mathematical foundations and framework for controlling and optimizing complex systems by developing data-driven machine learning and artificial intelligence. In US Department of Energy (DOE) mission areas, the analyses, simulations, and optimizations of these complex systems have been the focus of numerous past research efforts.
We recognize the fundamental challenge of complex system modeling and control, which is that high-fidelity ab initio models can be prohibitively expensive to construct. This limitation is not necessarily due to a lack of fundamental understanding of the underlying natural phenomena but due to practical constraints such as the difficulties in instrumenting the complex systems to collect relevant data, the need to resolve inherent uncertainties in the models (e.g., due to unmodeled physics), and the loss of observability due to excessive exogenous noises. The general applicability of the current solutions is hampered by the ad hoc nature of the approaches and the lack of a coherent theoretical framework.
We aim to develop a unified theoretical foundation based on probabilistic graphical models (PGMs). PGMs are a rich mathematical formalism with theoretical connections to many tasks in machine learning, such as uncertainty quantification and reinforcement learning. To leverage PGMs for the decision and control of complex systems, many open research questions remain, including model structure determination, model parameter estimation, and the foundational issue of analyzing uncertainties.
To address these challenges, our research efforts are organized into four interdependent technical thrusts: (i) model construction, (ii) uncertainty quantification, (iii) decision and control, and (iv) continual learning. The focus of the first two thrusts is to develop theories and methods for construction of PGM-based complex system models based on observational data under multiple sources of uncertainty.
The goal of the decision and control thrust is to develop a control mechanism for complex systems with uncertainty and limited observability by using techniques such as reinforcement learning. The continual learning thrust copes with the time-varying nature of the underlying dynamics of complex systems. A fifth thrust, application demonstration, is organized as a cross-cutting effort to develop the strategy for general applicability and validation of the research outcomes, and to conduct proof-of-concept demonstrations on applications from DOE mission areas, including building energy efficiency, reactor power control and science user facility control.
DnC2S bring together multidisciplinary researchers from Oak Ridge National Laboratory; Pacific Northwest National Laboratory; the University of California, Santa Barbara; and Arizona State University. We believe that the success of DnC2S will not only advance our understanding of complex systems from a theoretical perspective but will also significantly impact DOE scientific missions.